Revolutionizing COVID-19 ARDS prediction: a deep learning model for critically ill patients
Renji #Renji
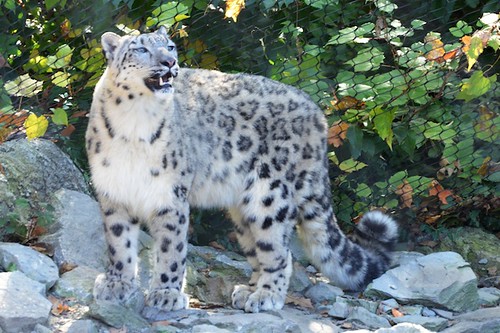
In a recent study published in Frontiers in Medicine, a group of researchers developed and evaluated a deep learning model for predicting coronavirus disease 2019 (COVID-19) acute respiratory distress syndrome (ARDS) in critically ill patients based on their clinical data and computed tomography (CT) images.
Study: A deep learning model for predicting COVID-19 ARDS in critically ill patients. Image Credit: PopTika/Shutterstock.com Background
COVID-19 is caused by severe acute respiratory syndrome coronavirus 2 (SARS-CoV-2) and can lead to severe pneumonia. About 33% of patients are at risk of developing severe symptoms with high mortality. Severe SARS-CoV-2 infection can result in ARDS with pneumonia-like symptoms.
Current ARDS management has limitations, shifting the focus to prevention by identifying high-risk patients. Early prediction of COVID-19 ARDS is crucial, and artificial intelligence (AI) offers promise in this area. AI’s ability to handle vast data aids disease diagnostics, prognostics, and personalized treatment. Combining CT scans and clinical data can enhance the precision of predicting COVID-19 ARDS, improving patient outcomes.
About the study
The study examined patients admitted to the intensive care unit (ICU) of Shanghai Renji Hospital between April and June 2022. Patients aged 18 and above diagnosed with COVID-19 ARDS were included. Those diagnosed with ARDS on the first day of admission, with over 20% missing clinical data or without CT scan results, were excluded. The study was approved by the institutional Ethics Committees, and patient consent was not required.
COVID-19 ARDS was diagnosed based on clinical history, epidemiological contact, a positive SARS-CoV-2 test, and the Berlin definition of ARDS. A set of chest clinical data and CT images were collected post-admission.
The data comprised comorbidity conditions, demographic information, onset symptoms, respiratory support methods, vital signs, aeration variables, inflammation tests, biochemical tests, routine blood tests, lymphocyte subset tests, blood coagulation tests, and cytokine profile tests.
Statistical analysis was performed using Python, and R. Categorical variables were compared using chi-square or Fisher’s exact test, while continuous variables were compared using the Mann–Whitney U-test. Multivariate logistic regression identified independent risk factors linked with COVID-19 ARDS.
Four machine learning algorithms were used to establish predictive models for COVID-19 ARDS. The training cohort was divided into five partitions, with four-fifths used for training and the rest for validation. Hyperparameters were fine-tuned to avoid overfitting.
CT slices were manually labeled and classified as normal or abnormal. A deep learning framework based on visual geometry group (VGG)-16 was used to label the remaining CT slices. The auto-labeled CT slices were then used to predict COVID-19 ARDS based on CT images.
Two prediction models based on clinical data and CT images were integrated using the penalized logistic regression algorithm. The performance of the integrated model was evaluated in the test cohort using the receiver operating characteristics (ROC) curves and confusion matrices. Calibration plots were also used to assess the predictive performance of all models.
Study results
The study enrolled a total of 103 patients, of whom 23 (22.3%) developed COVID-19 ARDS. A total of 3,187 chest CT images were obtained from the patients, with 690 CT slices from COVID-19 ARDS individuals and 2,497 CT slices from non-COVID-19 ARDS patients. Among these, 897 CT slices from 30 patients were manually classified as normal or abnormal.
The authors reported that after conducting multivariate logistic regression analysis, five independent risk factors associated with COVID-19 ARDS were identified: age, partial pressure of oxygen in arterial blood (PaO2)/ fraction of inspiratory oxygen concentration (FiO2) ratio, C-reactive protein, total T lymphocyte count, and interleukin (IL)-6.
Further, four machine learning models were developed to predict COVID-19 ARDS: logistic regression, random forest, support vector machine, and extreme gradient boosting (XGBoost).
The ROC curves for all the models revealed that the XGBoost model had the highest area (AUC = 0.94), surpassing the logistic regression model (AUC = 0.82), support vector machine model (AUC = 0.77), and random forest model (AUC = 0.92).
The authors conducted the Delong test to compare the XGBoost model’s AUCs with the other three models, resulting in significant differences (XGBoost vs. logistic regression model, P < 0.001; XGBoost vs. support vector machine model, P < 0.001; XGBoost vs. random forest model, P = 0.002). Based on the findings, the XGBoost model was selected as the most effective machine learning model for predicting COVID-19 ARDS.
A classification convolutional neural network (CNN) model based on individual CT images was trained using 897 manually labeled CT slices. The model achieved an AUC of 0.99, correctly distinguishing between normal and abnormal CT slices.
An integrated deep learning model, combining the XGBoost model and the CNN model, was developed. The AUC values for the XGBoost model, CNN model, and integrated model were 0.94, 0.96, and 0.97, respectively. The calibration curve indicated good agreement between the predicted probabilities and the actual outcomes.
To sum up, the results showed that the integrated deep learning model demonstrated higher accuracy in predicting COVID-19 ARDS compared to the individual models based on clinical features or CT images.