Beyond The Hype: Four Ways Artificial Intelligence Is Transforming Healthcare
Artificial Intelligence #ArtificialIntelligence
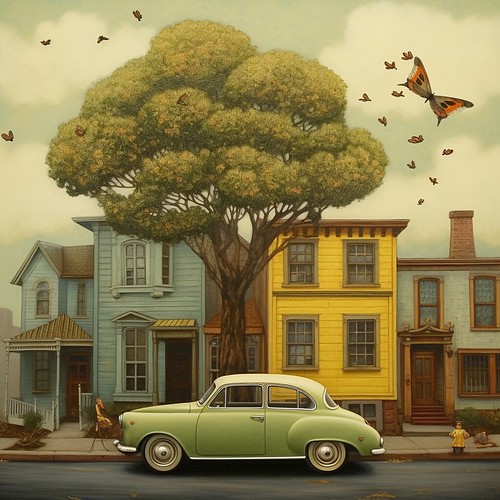
From improving patient outcomes and easing clinicians’ workloads to advancing clinical trials and predicting supply shortages, AI is supporting better, smarter, faster healthcare.
These days, it seems like everyone is talking about ChatGPT, and more broadly, the promise of artificial intelligence (AI) to enhance how we live, work and play. With all the hype surrounding AI, how do we determine where the technology can be of most benefit? How do we know it’s delivering real value?
getty
One industry where AI is proving to have positive application is healthcare – where the pace of change has left providers no choice but to augment intelligence with machines to deliver better outcomes.
Today, healthcare providers are operating in an overloaded environment. A new scientific or research study is released every 26 seconds. If a clinician read and retained two of these articles every single night, at the end of a year, they’d still be about 1,000 years behind the current evidence.
AI has tremendous potential, including the ability to sift and parse through all that data, glean critical insights, build predictive models, improve diagnosis and treatment of diseases, optimize care delivery, streamline tasks and workflows, and more.
Here are four ways AI is transforming healthcare and insights on how we can benefit.
Enabling Higher-Quality Patient Care
Of the many use cases for AI in healthcare, the biggest drivers center around empowering providers to deliver better, more cost-effective care for patients. AI-powered clinical decision support (CDS) tools can aid in developing accurate, appropriate and actionable diagnostic or treatment recommendations – an important outcome considering overtreatment/undertreatment of care can account for up to 30 percent of healthcare costs. CDS can also help reduce clinician burnout, enhance the clinician experience and increase productivity.
CDS tools deliver real-time alerts and relevant analytics to help guide and influence physician decisions. The technology relies on machine learning (ML) to embed alerts in electronic medical records and integrate them into routine workflows, so providers receive alerts for the right patient at the point of care.
Driven by evidence-based, clinician-designed logic, alerts are only triggered if the patient meets a specific profile and only provide recommendations that are relevant to the clinician who has final decision-making authority.
AI proof point: CDS utilization saved ≈$1K per patient encounter and yielded better patient outcomes, including shorter lengths of stay, a lower probability of 30-day readmissions and a lower probability of complications.
Revolutionizing Clinical Research and Discovery
AI is improving clinical trials – supporting diversity in recruitment and innovation in operations to ensure all populations have equitable access to breakthrough medical technology faster than ever before. Real-world data coupled with ML and natural language processing (NLP) can help providers enhance clinical trial design, feasibility and execution; reach underrepresented populations; compress study timelines; and improve life cycle management.
In addition to clinical trials, AI is helping to advance early disease identification and intervention. Data, combined with NLP, which can read and interpret more than 2 million records per hour, is being used to uncover subtle details that can point to a more serious diagnosis, often found in the unstructured narrative or clinician notes in patient charts that aren’t easily mined in mass.
These details help to identify the risk factors and clinical signs and symptoms most predictive of subsequent disease development and get to a definitive diagnosis faster.
AI proof point: NLP was utilized to identify patients with incidental pulmonary nodules (IPNs) to flag for intervention before potential lung cancer progression, with roughly 152,000 patients caught early.
Building Healthcare Supply Chain Resiliency
Historically, the healthcare sector lacked the ability to predict when a product might become short. Longitudinal visibility across the supply chain, where providers can see demand signals, point-of-use information and supplier resiliency metrics, is vital to accurately manage forecasting and predict supply shortages that can compromise quality patient care (think of the COVID-19 pandemic where shortages of personal protective equipment left clinicians, nurses and other healthcare workers ill-equipped to care for patients).
Predictive models driven by data shared between suppliers and providers combined with ML can help provide this much-needed longitudinal visibility. Armed with these insights, suppliers can anticipate increased demand for planning production, managing inventory and preventing shortages – in and out of a pandemic.
Providers receive real-time notifications when purchased products are at risk of shortages. The most innovative predictive systems can pinpoint product shortages with greater than 90 percent accuracy and automatically recommend clinically approved equivalent products – saving staff precious time and minimizing supply chain disruptions for steady supply and patient care continuity.
AI proof point: One health system used AI-driven predictive models to proactively address hundreds of potential shortages before patient care was impacted.
Optimizing the Healthcare Workforce
Staffing shortages in healthcare continue to create challenges, and if today’s trends continue, one study projects more than 6.5 million U.S. healthcare professionals will permanently leave their positions by 2026, while only 1.9 million will step in to replace them – leaving a national industry shortage of more than 4 million workers.
Workflows automated with AI capabilities can help extend scarce labor resources, reduce work fatigue and burnout, and enable operational and cost efficiencies. Prior authorization (PA) is one workflow ripe for automation. With today’s PA process, providers must submit specific paperwork to payers to obtain approval of a healthcare service or medication before care is administered – a time-consuming, manual task that may cause care delays and put patients at risk.
Cloud-based, ePA solutions that leverage AI-powered tools can help streamline the burdensome PA process by enabling quick, efficient decisions so treatment plans can move forward right away.
AI proof point: One study found that 71 percent of providers who implemented ePA reported faster time to patient care. Utilizing ePA also reduced the time between submitting a PA request and receiving a decision from the payer by 69 percent.
Where do we go from here?
It’s clear the use of AI in healthcare holds immense potential and promise for all of us, ushering in a new era filled with advancements in diagnostics and treatments, therapeutic discovery and clinical research, supply chain resiliency, and a host of operational and administrative efficiencies never before possible.
As AI rapidly evolves, we must continue to look beyond the hype that surrounds it and navigate the changing landscape cautiously to ensure the responsible integration of these technologies into our healthcare ecosystem for both providers and patients.
For more information, get your complimentary copy of The Pulse of AI: The Future of Technology in Healthcare.