Data Analytics
Analytics #Analytics
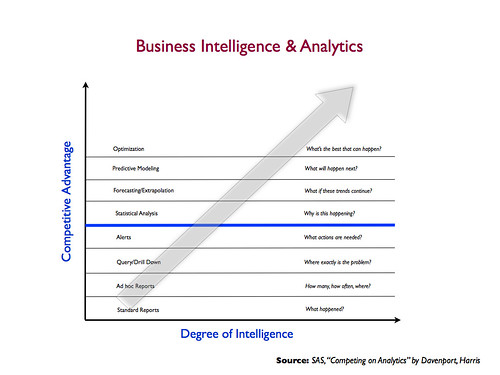
Thought Leadership Florian Zettelmeyer and Eric Anderson don’t worry about how to advance data science in the world of business. “It’s very advanced and good at what it does,” said Zettelmeyer, who, together with Anderson, is an Academic Director for Kellogg Executive Education’s Leading with Advanced Analytics and Artificial Intelligence program. “But in order to really take advantage of it, you now have to adapt the business processes to know how to operate in this new environment.”
That need to adapt is a core challenge for leaders trying to leverage everything these powerful tools have to offer, agreed Anderson, who co-authored the recently released Leading with AI and Analytics: Build Your Data Science IQ to Drive Business Value with Zettelmeyer. “That needs to change so that we can adapt to what’s implied by the AI, the machine learning, and the model saying, ‘Hey — do something different.’ The outcome is that you should change the way you’re making decisions, but a human has to agree with that.”
And humans are, well…only human. That’s where the real challenges arise. “Everything is done in this digital environment,” Anderson said. “Inject a person into the equation, and you start to have problems. The interface between science and people creates all sorts of complications. The prioritization of problems has to be a choice by a person. Do you agree or disagree with the machine’s recommendation? If you agree, what are the activities that have to change for you to support making decisions differently? Turning a data science recommendation into an ongoing process is really, really hard.”
Zettelmeyer and Anderson pointed to the retail environment to illustrate the type of problem that can arise once a human being is added to the situation. It’s one thing, they said, to determine the negative effect of running out of stock on a given item. It’s quite another to convince someone to make a change in order to solve that problem. “If you really want to have an impact with analytics, the difficult part isn’t to make the calculation,” Zettelmeyer stressed. “You have to ensure the calculation makes its way into the systems that humans use to make reordering decisions.”
Therein lies the rub, Anderson noted, adding there are often two ways a person falls short in implementing an AI-recommended solution. “The first is that they completely ignore what you say. Another is they agree to do it once, and you see only a short-term benefit. How do you embed it in the day-to-day decision-making as a process over the long run? It doesn’t do you much good if you quickly go back to the old way of working.”
Another example of difficulties introduced by human decisions is the reluctance to experiment in a scientifically meaningful way in order to learn from mistakes. One can make an honest error and learn from it, Zettelmeyer and Anderson said, but to really excel you must purposefully introduce “errors,” deviations from what you think is optimal, so that you know for sure what is best. For instance, a retailer carries three sizes of battery—small, medium and large. Someone in inventory forgets to order the medium, and the result is that carrying only the small and large sizes is more profitable. Too many managers would continue with those two sizes, but there’s no way of knowing if that’s the best choice without testing other combinations.
“You never answered the question of what you should carry,” Anderson said. “That might be the right thing for the moment. But there is an ungodly number of assortments to try, and you haven’t tried them. You have to intentionally do the wrong thing to get the right data. It’s the intentional part of it that’s so hard for everybody. It’s expensive to make mistakes, but this is what enables learning.”
“It’s easy to say you reward experimentation,” Zettelmeyer added. “But it’s difficult if your compensation structure looks strictly at profit and loss.” The answer is to set different goals. “Make the goal running that experiment in addition to maximizing a financial reward. Then you’ve come closer to solving the problem.”
“At a high level, it’s about changing your culture,” said Anderson. “You need to collaborate internally and set benchmarks for shared success. It calls for new ways of doing things and making evidence-based decisions that cut across the enterprise. “That’s hard. Everybody quickly reverts to what worked before.”